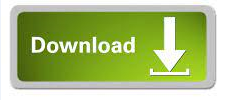
In: Proceeding 13th IEEE international conference on automatic face & gesture recognition. pp 1–30Ĭao Q, Shen L, Xie W, Zisserman A (2018) VGGFace2: a dataset for recognising faces across pose and age. IEEE Trans Inf Forensics Secur 14:1181–1193īounareli S, Argyriou V, Tzimiropoulos G (2022) Finding directions in GAN’s latent space for neural face reenactment. īoroumand M, Chen M, Fridrich J (2018) Deep residual network for steganalysis of digital images.
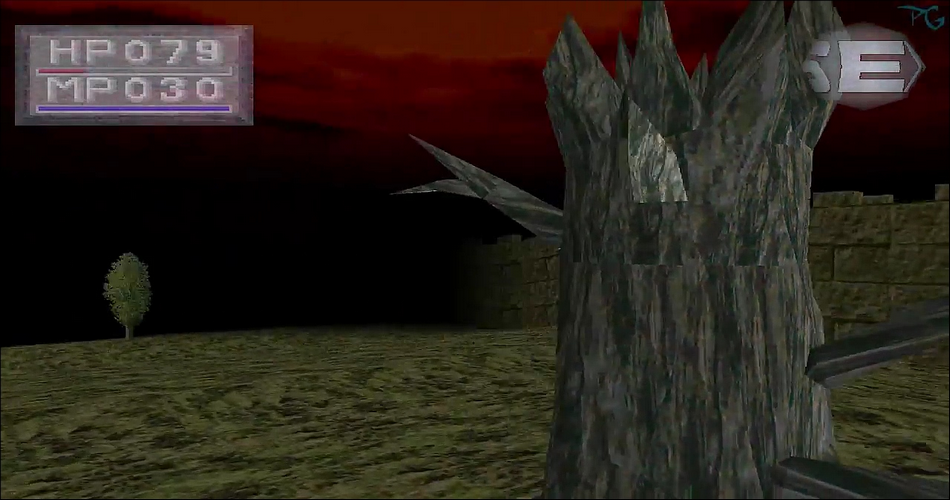
In: Proceedings of the IEEE Conference on Computer Vision and Pattern Recognition Workshops (CVPRW). We have compared the proposed approach with existing state-of-the-art systems and show that our method achieves qualitatively and quantitatively better results.Īgustsson E, Timofte R (2017) NTIRE 2017 challenge on single image super-resolution: dataset and study. The face blending network is used to blend two faces seamlessly. We propose a steganography-based GAN model and used benchmark datasets such as Flickr-Faces-HQ (FFHQ), IMPA-FACE3D, FaceForensics++, and CelebFaces Attributes (CelebA) facial datasets in the experimentation. We have derived a Generative Adversarial Networks-based approach to face re-synthesis and re-enactment that adjusts for facial expressions and pose. A comparative study has been conducted that showcase the drawbacks of existing literature and motivated their work. The facial re-enactment GAN (FRe-GAN) technique and qualitative and quantitative results have been presented over various datasets.

The preprocessed standard datasets create steganographic datasets for facial re-enactment purposes. This paper presents a technique for hiding secret messages in images while transferring them over a network using steganography.
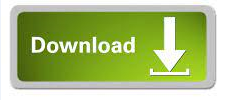